Explore the future of data architecture with insights on data mesh, AI-driven infrastructures, and data lakehouses.
The world of data architecture continues to evolve, with innovative approaches transforming how organizations can manage and utilize their data. From decentralization to AI-driven advancements, businesses are exploring new frameworks to stay competitive and agile. In this blog, we delve into three key trends shaping the future of data architecture: the rise of data mesh and fabric, the push for AI-driven infrastructures, and the evolution of data lakehouses. These breakthroughs promise to redefine scalability, accessibility, and efficiency in data operations. But are they ready for widespread adoption?
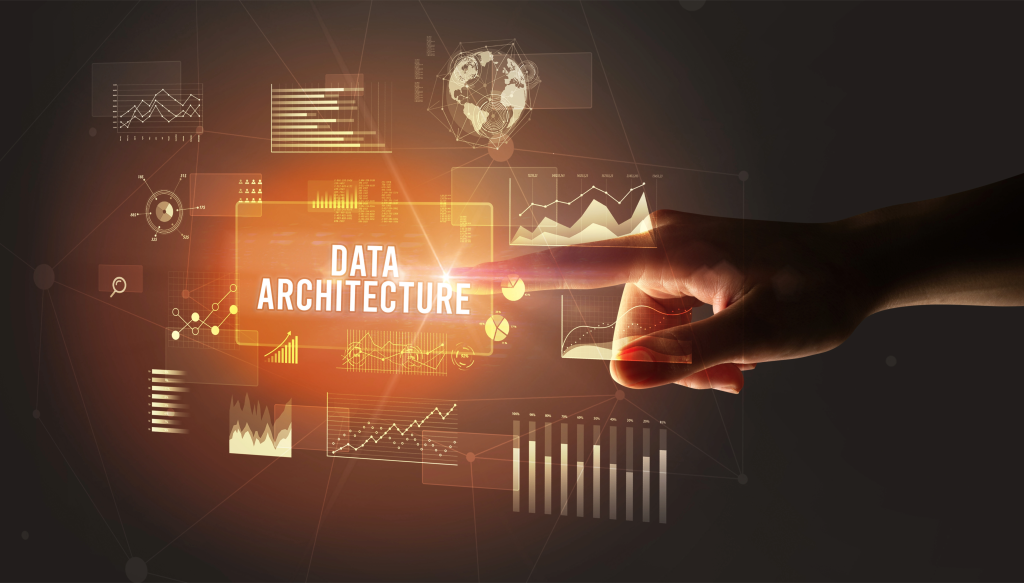
Rise of Data Mesh and Data Fabric
Data mesh and data fabric are gaining traction as transformative approaches to data architecture. Data mesh emphasizes decentralization and assigns ownership of data to individual domains within an organization. Meanwhile, data fabric provides an integrative layer, connecting diverse data sources for seamless access and analysis.
While promising, these approaches remain in their infancy. Organizations adopting them have encountered shortcomings, such as complexities in implementation and gaps in existing tools. By developing workarounds and addressing these pain points, we’re beginning to see their potential to combat data silos and improve scalability. As these frameworks mature, they could redefine how we think about enterprise data management. Keep an eye on how these innovations develop.
Increased Adoption of AI-Driven Data Architecture
The biggest challenge for many organizations in adopting operational AI lies in outdated or inadequate data infrastructure. To succeed, operational AI requires a modern data architecture that provides the flexibility and visibility needed to simplify data access, break down silos, and make data more actionable.dat
These advanced architectures support the integration of diverse data sources and formats, creating a cohesive framework for efficient data operations. However, many companies are still wrestling with data deficiencies, particularly in data quality and governance. Additionally, managing unstructured data has proven to be a significant challenge as organizations strive to extract meaningful insights. Addressing these issues through continuous adaptation and investment in scalable, robust infrastructures is critical to building AI-ready architectures capable of delivering impactful results.
The Evolution of Data Lakehouses as a Unified Platform
Data lakehouses combine the scalability of data lakes with the performance of data warehouses, offering a unified platform for storing and processing both structured and unstructured data.
By simplifying data ecosystems, lakehouse technologies could streamline analytics and operational workloads. Organizations are increasingly leveraging this approach to balance flexibility with performance, making data more accessible and actionable across the enterprise. However, shortcomings remain, with security and integration at the top of the list. As the technology continues to mature, lakehouses have the power to unlock new possibilities for data-driven innovation.
Conclusion
As organizations continue to navigate the complexities of a data-driven world, these emerging trends in data architecture are paving the way for a more streamlined and powerful future. Whether it’s decentralizing through data mesh, optimizing to support AI, or unifying with lakehouses, each approach brings unique opportunities to address longstanding challenges.
Stay tuned as these technologies mature and evolve into practical solutions. Until then, we must remain diligent in evaluating whether the technology supports the use cases we are trying to solve. Only by aligning innovation with practical needs can we ensure these advancements truly deliver value. In the meantime, leveraging tried-and-true, cost-effective methods such as an operational data store (ODS) or a data warehouse can provide stability and reliability while new technologies develop.
Written By:
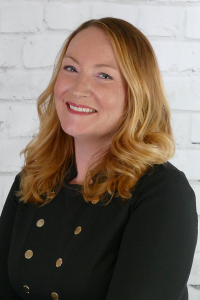
Morgan Duckworth, Practice Leader – Business Systems and Data Architecture, Data Strategy Group