By leveraging IoT sensor data, this solution enhanced predictive maintenance, cut costs, and improved service reliability.
Energy control systems are designed to help plant operators manage their operations to ensure the safe, reliable production of energy. Plants with lower operating costs and high availability meet their native load and are dispatched more frequently to participate in the wholesale market, leading to improved profits. The need to balance power distribution, reduce operating costs, reduce emissions, and maintain high availability are all dependent on an effective asset management program, and data.
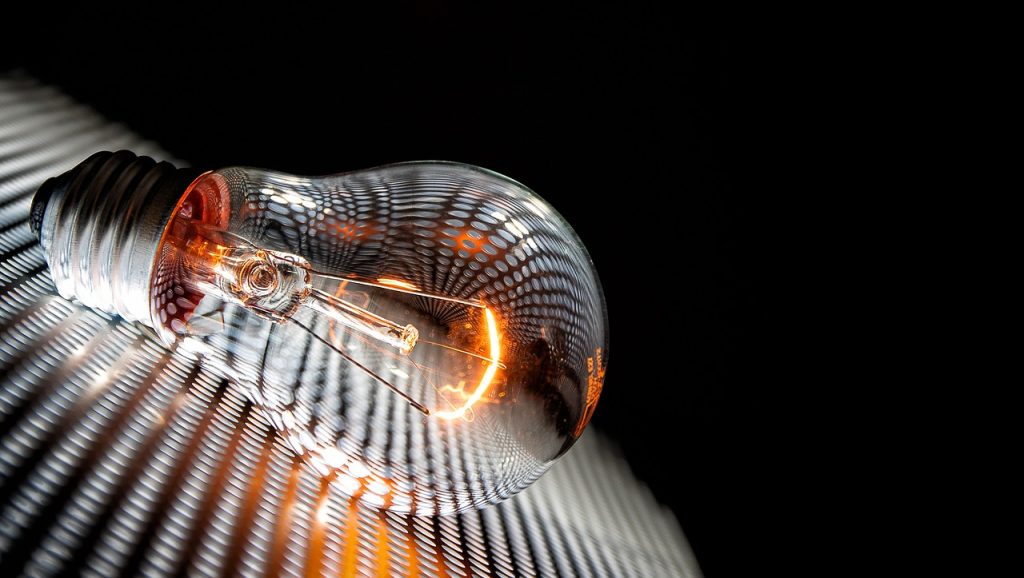
Our client’s control system had many IoT sensors collecting high frequency time series data that could be analyzed. The challenge was getting access to that data to do the analysis. The process involved manually extracting and loading this sensor data into individual Excel spreadsheets. This was time-consuming and provided limited ability to analyze such a large volume of data which was necessary to see the patterns that may indicate a system issue. It wasn’t helping to schedule maintenance more effectively and did little to reduce unplanned plant outages.
To address these challenges, we developed an automated solution to streamline the data extraction and analysis process. We created an on-demand job capable of extracting data from all IoT sensors and loading it into a new analytics platform.
We developed visualizations that mirrored the familiar Excel graphs used by the client’s team, ensuring a smooth transition to the new system. The new platform introduced the capability to cross-correlate data from multiple sensors, providing deeper insights into the system’s performance and potential issues.
The implementation of the automated data extraction reduced a multi-day manual process to just a 5-minute job. This significant time saving allowed the team to focus more on data analysis rather than data management. Furthermore, the ability to extract data more frequently meant that potential system issues could be identified and addressed earlier, reducing the risk of unplanned outages and downtime.
The new capability to analyze correlations between various sensors provided a more comprehensive understanding of the system’s overall health, enabling more precise predictive maintenance scheduling. This not only improved the plant’s operational efficiency but also reduced costs associated with unexpected repairs and downtime.
In conclusion, the integration of an automated analytics platform and advanced data visualization tools transformed the client’s approach to predictive maintenance. By leveraging IoT sensor data more effectively, the client could optimize plant operations, reduce downtime, and improve overall service reliability. This case highlights the critical role of modern data analytics and automation in enhancing the efficiency and reliability of energy systems.
Written By:
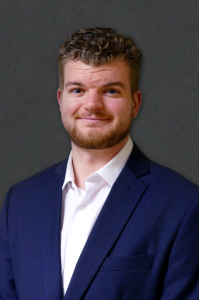
Cody Krietemeyer, Practice Leader – Data Engineering, Data Strategy Group