The average data scientist spends 70% of their time procuring and cleaning data.
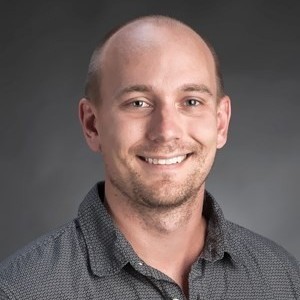
Will Hawkins, PhD – Data Scientist, Data Strategy Group | Connect with Will on LinkedIn
If you work around data scientists or analysts, you’ve likely heard the phrase “dirty data”. Dirty data often slows down analysts and scientists in their initiatives to drive value through analytics and automation and can even make these initiatives fail altogether. So, what is dirty data? How can a collection of bits stored in a server possibly be dirty? Here is a non-exhaustive list of examples of dirty data described by a brief example. There’s a great chance your organization has all of them present!
- Incomplete Data: Let’s say that your B2B sales team hasn’t classified the customers they sell to by size. This means that data scientists and data analysts can’t segment their analyses by company size unless they do that classification on their own.
- Duplicate Data: Duplicate data strains IT infrastructure budgets (storage and compute costs) and requires the data scientist or analysts to remove duplicate records from their analyses.
- Incorrect Data: Incorrect data occurs when a field contains data outside the valid range of values. For example, the number 13 would be an incorrect value for the field “month”.
- Inconsistent Data: Inconsistent data is defined as redundant data that doesn’t match. For example, many organizations have customer information in multiple systems that may have the same field names but are calculated using different methods or updated at differing frequencies.
So, who cares? Dirty data makes your analytics and automation initiatives take longer, which means loss of momentum and soaring costs. The average data scientist spends 70% of their time procuring and cleaning data. This time doesn’t drive value back to the organization and reduction of this waste is sure to improve your analytics and automation processes. If you’re interested in learning more about how to systematically cleanse your data, we would love to partner with you.