Data architects utilize dimensional models to consolidate data from multiple sources in a data warehouse for operational decision-making and leverage, whether traditional or cloud-based.
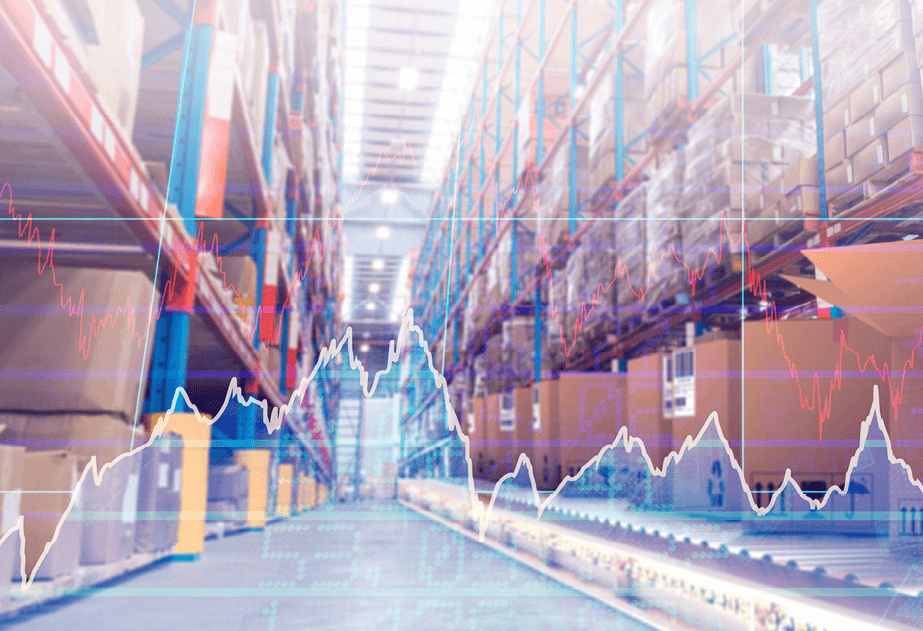
Wide-leg jeans, cropped shirts, and platform sandals have recently made a comeback. Ask my teenage daughters, and they’ll tell you how cool these things are. But you know what else is cool again? Data warehouses. Just do a simple internet search, and you can read countless articles about modern data warehouses, data warehousing methods, or data lakehouses. You’ll quickly realize that a data warehouse and its methodologies are still relevant – maybe even cool after all these years. Why is this? Why haven’t all the “data warehouse killers” succeeded? Let’s take a closer look.
Dimensional Data Modeling and the Data Warehouse
Over 25 years ago, Ralph Kimball introduced the concept of dimensional data modeling. Organizations of all sizes have adopted this modeling method to present data in a data warehouse to support analytical decision-making for business users. Dimensional data modeling utilizes a star or snowflake schema to represent data in a structured and intuitive way. The star schema separates business data into facts that hold measurable, quantitative data about a business process or event and dimensions that are descriptive attributes related to that fact data. The star schema simply contains a fact table surrounded by dimension tables, while a snowflake schema is an extension of this and can contain additional layers of dimension tables.
A data architect can deconstruct the organization’s reporting needs into its underlying business processes and events in order to define the facts and dimensions required. By designing a dimensional model based on business processes, the end result is often more resilient than the ever-changing requests for information. A data warehouse enables organizations to consolidate data from multiple sources in this manner and ensures they own their data for the long term. This provides a valuable asset that can be leveraged over time to drive business growth and success.
Fast forward to today. The modern data warehouse resides in the cloud but uses the same design methodology of traditional data warehouses: integrating data from many different sources into a highly accessible format used for reporting and operational decision-making.
“The modern data warehouse resides in the cloud but uses the same design methodology of traditional data warehouses: integrating data from many different sources into a highly accessible format used for reporting and operational decision-making.”
The Demise (or Lack) of the Data Warehouse
In the mid-2010s, the concept of big data and data lakes entered the business intelligence landscape, and many declared that traditional data warehousing was dead. The data lake allowed organizations to file information without worrying about its structure while taking advantage of cheap cloud memory and storage. Data lakes, by design, only put data from different sources into one place mainly for data science use; they do not build a unified layer of data by integrating relevant sources together. Analysis across multiple sources proved tricky in the data lake; thus, the data warehouse lived on to support the organization’s analytical needs.
Next, the data lakehouse emerges. This concept is said to take the best of the data lake and data warehouse and combine them in a cloud-based environment. Bill Inmon even repurposed his 1992 book, Building the Data Warehouse, to write Building the Data Lakehouse in 2021. He explains that the unique ability of a lakehouse is to manage data in an open environment, blend data from all parts of the enterprise, and combine the data science focus of a data lake with the end user analytics of a data warehouse. While data lakehouses are a good solution for organizations where data is used by both business professionals and data science teams, the technology is still immature. The data lakehouse has not caused the demise of the data warehouse just yet. However, there are many lakehouse vendors working diligently to deliver a solid product built on cloud technology.
It is also worth mentioning that data fabric is sometimes marketed as a replacement for a data warehouse. But note that data fabric is a design concept and a data management architecture that actually uses data warehouse methodology to logically combine data across environments through the use of intelligent and automated systems.
Conclusion
Could your streaming data from IoT devices be filed in a data lake? Yes. Could you place big data alongside other data sources in a data lakehouse? Absolutely. Should organizations look at how data fabric concepts and architecture can allow their decision-makers to have insights on the go? For sure. There are many use cases for each of these technologies and concepts. However, none are yet able to kill the data warehouse. This begs the question – does your organization struggle to keep up with the demands for information or figure out how to combine data from disparate systems effectively?
Consider a dimensional model to present data for consumption in your organization via a cloud data warehouse: it is capable of handling a massive amount of complex data, can instantly be scaled up or down based on business needs, can perform rapid advanced analytical queries, and contains limited infrastructure setup costs. The modern data stack is changing rapidly, and technological advancements are made daily. However, I will bet that data warehousing concepts and methodologies will continue to be cool for a while longer. I will also bet that the same won’t hold true for the wide-leg jeans you sported to work this morning.
Written By:
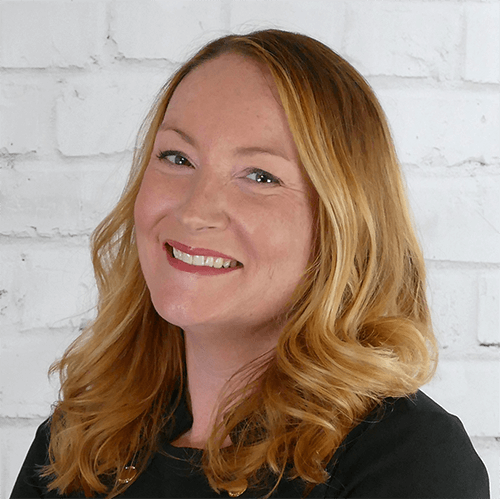
Morgan Duckworth
Practice Leader, Data Architecture
Data Strategy Group